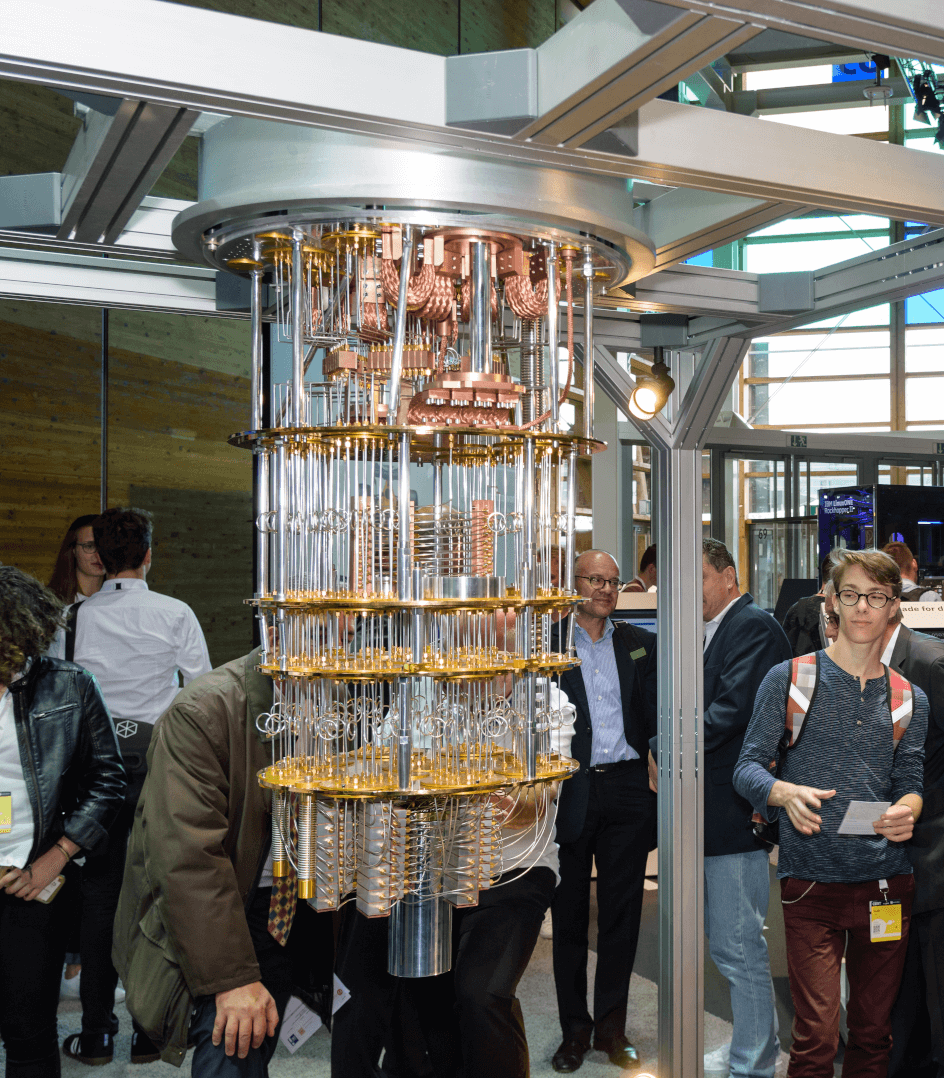
Quantum computing, the latent revolution, a focus of innovation for Izertis
Quantum computing will mark a new era thanks to its capacity to solve problems that classical computers are incapable of addressing, promising a computational advantage over the classical approach when it comes to solving challenges that are associated with huge volumes of combinations. In this way, they can tackle problems whose complexity grows exponentially as the scale of the problem to be solved increases, that is, as the number of variables that must be considered to solve them grows.
Despite the immaturity stage of classical-quantum hybridization, the urgent need for its implementation foresees a strong demand in the short and medium term from the very moment in which the minimum computational advantage is achieved with respect to the pure classical paradigm. Computer security, biomedicine and mobility are some of the fields that could be revolutionized by advances in quantum computing.
Our company, aware of this potential, of its disruptive role and of the impact it will have in many areas and in a very notable way in the capacity of massive calculation and in communications with secure encryption, has started in the last three years a path of investigation and application of this new paradigm. Our firm wants to stay close to this technology and take advantage of its growth to be able to participate in an emerging market where, due to its complexity and immaturity, obtaining a correct knowledge of the technology will allow it to position itself against multiple competitors.
At the end of 2019 our company began its foray into this field with the execution of the Q-COS project, in which hopeful results are being obtained that further drive our firm's interest in entering this technology. The general objective of this project was to simulate the behaviour of a quantum computer in a conventional computer, in such a way that quantum algorithms can be executed that provide an increase in precision with respect to the classical ones.
Q-COS draws conclusions that this technology offers a series of advantages compared to the tools currently used to solve certain complex problems (such as the optimization of investment portfolios), in a faster, more precise and efficient way.
At the end of 2020 our company started the QAI (Quantum Artificial Intelligence: Quantum Machine Learning in Finance) project that proposes a hybrid computing platform for solving complex financial problems that currently require intensive computational calculations (which can take up to days to complete) and in which a large number of dimensions or variables intervene to considerer to make the best decision.
Thanks to our firm' long experience in the field of Machine Learning under classical computing architectures, the technological challenges of the project have focused on the research and development of quantum Machine Learning algorithms that provide an advantage, in precision, with respect to the previous one.
At present, there are methods to detect and prevent fraud, customer segmentation or risk analysis. However, they are limited by the capabilities of current technology. QAI aims to cover the needs for technological knowledge and financial solutions in areas such as Fraud Detection, Risk Analysis or Customer Segmentation. The final objective is the research and development of a hybrid quantum-classical algorithmic architecture of Machine Learning models (QML), understood as Quantum Artificial Intelligence (QAI), aimed at generating ideas from automatic pattern recognition.
QAI will begin to deliver results and offer quantum artificial intelligence financial solutions that can be brought to a productive environment.
To carry out these projects, our company relies on its strategic alliance with IBM for access to cloud services offered by the Quantum IBM Researchers Program, which operatively integrates both computing paradigms (classical-quantum), offering a comparative dimension between both.